Introduction
This vignette gives a detailed account on the workflow of UniPath tool for analyzing single cell expression data and single cell open chromatin profiles in pathway domain. UniPath is a steadfast statistical method for getting important biological insights from single cells characterized in terms of pathway activity scores and studying temporal dynamics. UniPath is a scalable platform allowing pre-processing and analysis of thousands of single cells by exploiting heterogeneity among cells and uncovering biologically relevant pathways. UniPath can help users with accurate identification of cell types, signaling pathways and doublet cells. Besides these, the user can also perform clustering and pseudo temporal ordering of single cells in pathway space. This may allow the analysis of relevant pathways and genes on single cell lineage transitions or potency.
Installation
To install the package use following command.
library(devtools)
install_github("reggenlab/UniPath")
User can also install package using . tar.gz file. Click here to download UniPath_0.1.0.tar.gz file.
R CMD INSTALL UniPath_0.1.0.tar.gz
In case of installation issues, check for the dependencies associated with the package. They can be installed from the following links:
For other applications of UniPath, we suggest users to install DrImpute, ggplot2 and reshape2 packages. Also, we have used EmpiricalBrownsMethod package from R.
We have included codes and datasets for replicating results and figures from the paper. These can be downloaded from the Download button. To download user must provide details as follows: User id: reggen and password unipath@123
Usage and workflow
For single cell data analysis, UniPath package comes with a precompiled mouse and human null model data. Also, for transformation of single cell gene expression data into pathway or gene-set enrichment scores, the package contains pathway annotation and gene set marker files.
The analysis of single cells starts by loading following data files:
• Mouse/human null model data matrix
• Gene set maker file
• Gene expression matrix (Where genes should be in rows and samples/cells should be in columns). Ensure that genes are set as row names of the matrix.
We have used mouse lung data (GSE52583, Treutlein, Barbara et al.) consisting of 23007 genes and 194 cells for this tutorial. For example:
library(UniPath)
##Load all data files
data("mouse_null_model")
data("c5.bp.v6.0.symbols")
data("GSE52583_expression_data")
For tutorial purpose, in-built data is provided but users can also read or load their own input data files.
Conversion of gene expression FPKM value to P-value
Next, we transformed gene FPKM values to p-values using individual cell’s mean and standard deviation based on the assumption that non-zero FPKM value in cell follows log normal distribution.
##Converting mouse null data into p-values
Pval = binorm(mouse_null_data)
##Converting gene expression data into p-values
Pval1 = binorm(expression_data)
Combining of P-values
To take into account interdependency of p-values, we used browns method to combine p-values of genes in gene sets using combine() function for individual cells. User can define a threshold using thr option in combine() function to include those genesets having number of genes greater than the threshold value provided. Default value is 2. We are using pathway annotation file having 4436 gene sets for transforming data into pathway space.
##Combining of p-values for null model data matrix
combp_ref = combine(c5.bp.v6.0.symbols,mouse_null_data,rownames(mouse_null_data),Pval,thr=2)
##Combining of p-values for gene expression data matrix
combp = combine(c5.bp.v6.0.symbols,expression_data,rownames(expression_data),Pval1,thr=2)
Adjusting of P-values
To avoid unwanted effects of background housekeeping genes and for highlighting cell type specific gene-set activities, we adjust p-values using null model. This adjusted p-value matrix is referred to as pathway scores.
scores = adjust(combp,combp_ref)
This will return a list of three matrices one with absolute p-values, second one with raw adjusted p-values and third one is log transformed adjusted p-values.
Single cell ATAC-seq
UniPath transforms single cell open chromatin to pathway enrichment scores by highlighting cell type specific enhancers through normalization of profiles by global or local accessibility scores.
Global accessibility scores
To control for sequencing depth and dropout rate, firstly we highlight cell type specific enhancer by dividing scATAC-seq profiles by their global accessibility scores. For global accessibility scores computation, bulk ATAC-seq peaks have been curated. Using this combined/reference peak list, global accessibility scores are calculated for each genomic site based on the proportion of samples, the site was detected as open chromatin peak. Global accessibility scores for test dataset is calculated using global_access() function. For this tutorial, we have used human scATAC-seq keratinocytes differentiation data at three time points from GEO: GSE116248 study. Global accessibility scores for this dataset is calculated using global_access() function as shown in example below. User has to input genomic coordinate or peak file for this dataset along with the reference peaks list and human global accessibility scores.These data files are loaded from extdata folder of UniPath.
##First set up a working directory
setwd(system.file("extdata", package="UniPath"))
globalaccess = global_access("GSE116248_peaks.txt","reference_peaks_human.txt","global_accessibility_score_human.csv")
User can also perform imputation of scATAC-seq data as shown below. Imputation improves the quality of data, thus it's a user choice. For this tutorial, we have used Drimpute for imputation which is time consuming task. Therefore, imputed keratinocytes data is a part of this package. Raw count GSE116248_counts.csv file used for imputation can be downloaded by clicking Click here. Count file must be in .csv format and should contain genomic coordinates in rows and cells/samples in the columns as provided in this example.
imputed_count = drimpute("GSE116248_counts.csv")
Conversion of scATAC-seq profiles into pathway enrichment scores
UniPath provides two methods for normalization of scATAC-seq profiles in each cell, one is global accessibility score and other is using local accessibility score. After normalizing scATAC-seq profiles, peaks having high normalized counts are considered as a foreground set and all the peaks are used as a background set. Then, the nearest gene within default distance of 1MBp of the peak is considered for conversion into pathways enrichment scores using either hypergeometric test or binomial test. Nearest gene to the peak list can be calculated as shown in example below. User will require perl in the system to use this. This function takes four arguments: First is nearest gene perl script, second is peak list then human reference genome file and last one is output file name which you can specify along with the path of the folder where you want to save your output file. This is followed by system calling of perl script.
cmd = nearest_gene("nearestGenes.pl","GSE116248_peaks.txt","refseq-hg19.txt","GSE116248_foreground")
##system call
system(cmd)
This is followed by conversion of scATACseq profiles into pathway scores using runGO which requires pathway annotation file, background file available with the package, imputed or raw count matrix and foreground file.
Here we have kept a threshold of 1.25. Peaks above this given threshold value will be chosen to be in a foreground set. User can input their own threshold value. See the example below.
data("c2.cp.v6.1.symbols")
scores = runGO(c2.cp.v6.1.symbols,"background_human","GSE116248_imputed_count.csv",method=1,"globalaccess","GSE116248_foreground",promoters = FALSE,dist=1000000,threshold=1.25)
Above function will return list of two dataframes containing pathway scores based on hypergeometric and binomial test respectively. After conversion of scRNA-seq and scATAC-seq into pathway scores, user can perform number of downstream analysis to get biologically useful insights which are discussed in below sections.
In a similar way, we can tranform mouse single cell ATAC-seq profiles into pathway scores using mouse global accessibility scores.
Pseudo temporal ordering
In UniPath, for pseudo temporal ordering we first perform the initial level of classification of cells using hierarchical clustering approach on pathway scores matrix. Then in order to avoid wrong ordering of cells, we compute the top k nearest neighbor (KNN) index for each cell. And based on these indices and belongingness of the cells to same class as well number of times top KNN of a particular class is occurring in other class, we perform two levels of distance shrinkage for every cell pair. Using this shrunk distance matrix, minimum spanning tree is plotted. We have used 4 clusters for mouse lung data since there are 4 types of cells and k=5 for top k nearest neighbor computation.
Example of scRNA-seq data
##Performing hierarchal clustering on pathway score matrix
##User has to specify number of classes
distclust = dist_clust(scores$adjpvalog,4)
dist = distclust$distance
clusters = distclust$clusters
##Specifying number of top k nearest neighbor
index = index(scores$adjpvalog,5)
###getting cluster number for each of the KNN for individual cell
KNN = KNN(scores$adjpvalog,index,clusters)
##Two level shrinkage of distance matrix based on clusters and KNN
class = class1(clusters,KNN)
distance = distance(dist,class,clusters)
##Computation of MST on shrinked distance matrix
corr_mst = minimum_spanning_tree(distance)
User has to define the cell labels or groups along with the colors to be plotted on minimum spanning tree. It can be defined as shown below or user can input their cell labels.
##Plotting of MST
vertex_color = c("red","green","blue","yellow")
cell_labels = as.factor(c(rep("E18.5",82),rep("E14.5",44),rep("Adult",46),rep("E16.5",23)))
UniPath::mst.plot.mod(corr_mst, vertex.color = vertex_color[cell_labels],mst.edge.col="black",bg="white",layout.function="layout.kamada.kawai",v.size = 2, e.size=0.005,mst.e.size = 0.005)
legend("top", legend = sort(unique(cell_labels)), col = vertex_color,pch=20, box.lty=0,cex=0.6,pt.cex=1.5,horiz=T)
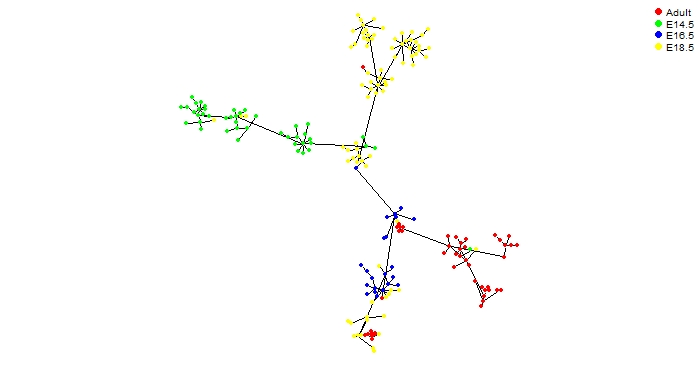
Considering mouse lung developmental data from Treutlein, Barbara et al., we performed pseudo temporal ordering to trace developmental hierarchy of lung from epithelial cells at 4 different stages.
Another example is of scRNAseq time course differentiation data of human embryonic stem cells from study Chu et al (GEO ID: GSE75748). We have included precompiled pathway scores of this study in the package, which can be directly used for performing pseudo temporal ordering as shown in example below.
This code can be used for replicating Figure 4b from the paper.
library(UniPath)
data("GSE75748pathwayscores")
distclust = dist_clust(GSE75748pathwayscores,6)
dist = distclust$distance
clusters = distclust$clusters
index = index(GSE75748pathwayscores,4)
KNN = KNN(GSE75748pathwayscores,index,clusters)
class = class1(clusters,KNN)
distance = distance(dist,class,clusters)
corr_mst = minimum_spanning_tree(distance)
vertex_color = c("yellow","Red","green","blue","black","magenta")
cell_labels = as.factor(c(rep("0h",92),rep("12h",102),rep("24h",66),rep("36h",172),rep("72h",138),rep("96h",188)))
set.seed(100)
UniPath::mst.plot.mod(corr_mst, vertex.color = vertex_color[cell_labels],mst.edge.col="black",bg="white",layout.function="layout.kamada.kawai",v.size = 3,e.size=0.005,mst.e.size = 0.005)
legend("topright", legend = sort(unique(cell_labels)) , col = vertex_color,pch=20, box.lty=0,cex=1,pt.cex=2,horiz=F)
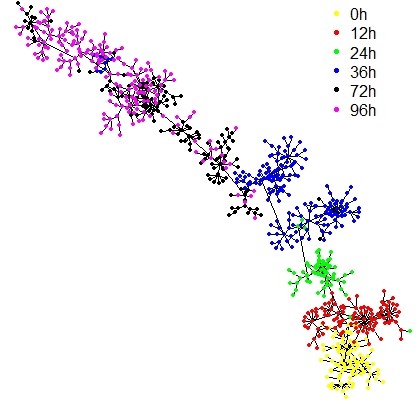
Example for scATAC-seq data
adjpva = -log2(scores$hypergeometeric+.001)
distclust = dist_clust(adjpva,3)
dist = distclust$distance
clusters = distclust$clusters
index = index(adjpva,5)
KNN = KNN(adjpva,index,clusters)
class = class1(clusters,KNN)
distance = distance(dist,class,clusters)
corr_mst = minimum_spanning_tree(distance)
vertex_color = c("red","green","blue")
cell_labels = as.factor(c(rep("0days",96),rep("3days",95),rep("6days",97)))
UniPath::mst.plot.mod(corr_mst, vertex.color = vertex_color[cell_labels],mst.edge.col="black",bg="white",layout.function="layout.kamada.kawai",v.size = 3,e.size=0.005,mst.e.size = 0.005)
legend("topright", legend = sort(unique(cell_labels)) , col = vertex_color,pch=20, box.lty=0,cex=1,pt.cex=2,horiz=F)
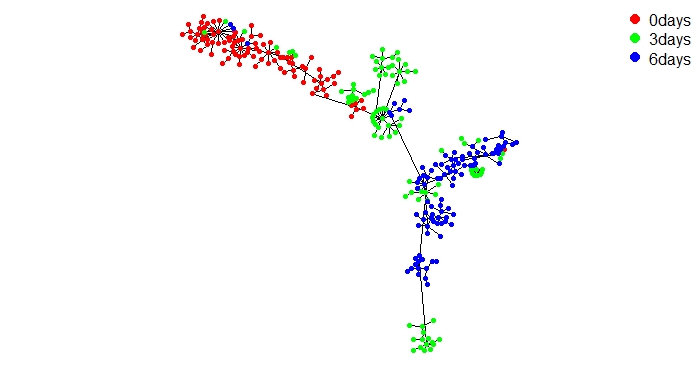
Above figure shows keratinocytes differentiation at 3 different time points.
Other beneficial features of using UniPath
Clustering and cell detection
Clustering and classification of cell types is a common task in analyzing single cell data. It helps in the annotation of new and rare cell type classes. Mostly this is done in gene expression space but UniPath introduced an approach to perform these tasks using pathway scores. Pathway scores helped us in cell annotation of unknown cell types along with detection of rare cell classes.
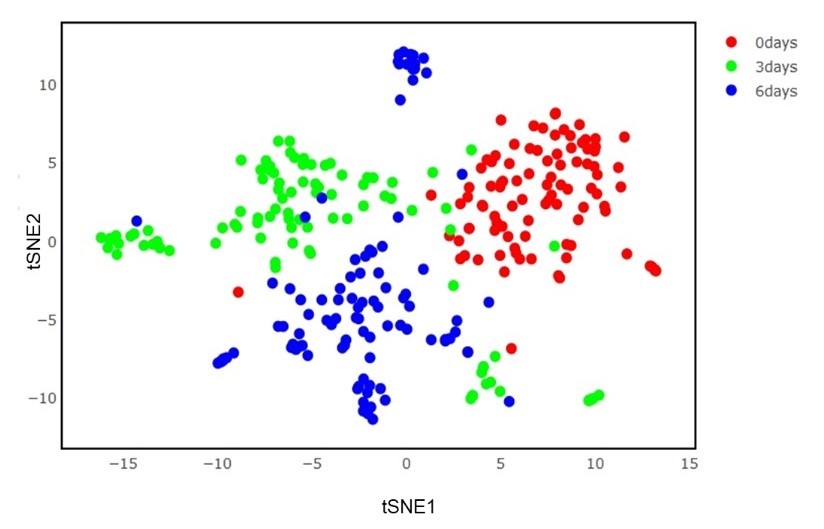
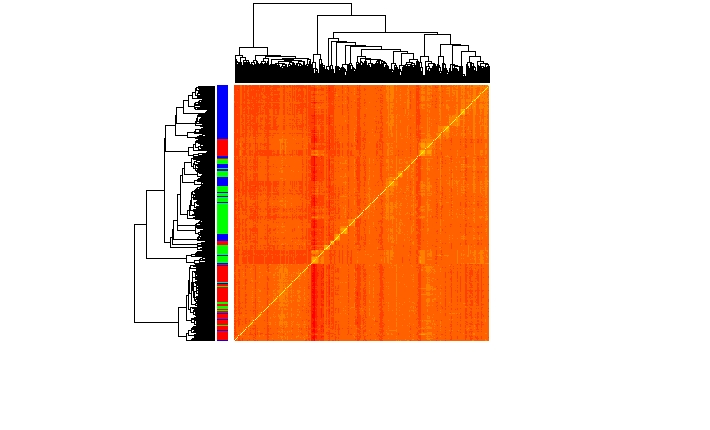
Above plots shows the separation of three classes in pathway space.
UniPath can also help in cell detection based on cell specific gene marker sets which are available in this package. Considering data from study id GSE81861, we have computed gene set enrichment scores using cell type specific marker file as shown in example below. User has an option to change the threshold value.
library(UniPath)
data("human_null_model")
data("GSE81861")
data("human_markers")
Pval = binorm(human_null_data)
combp_ref = combine(human_markers,human_null_data,rownames(human_null_data),Pval,thr=10)
Pval1 = binorm(data)
combp = combine(human_markers,data,rownames(data),Pval1,thr=10)
scores = adjust(combp,combp_ref)
In case of scATAC-seq profiles cell detection, human_markers_scATACseq.RData marker file can be used.
Differential pathway analysis
Generally, differential gene expression analysis is performed to distinguish between different cell populations but UniPath can help in finding differentially expressed pathways among different group of cells. It uses Wilcoxon rank sum test for allowing users to access statistically significant pathways among the group of cells. Here, we are using raw adjusted pathway scores of same mouse lung data transformed above for getting differential pathways between adult lung stage and rest of the cells in the example below.
##Use raw adjusted p-value data
data = scores$adjpvaraw
##define groups
group = as.matrix(c(rep(2,81),rep(2,44),rep(1,46),rep(2,23)))
wilx = temporaldif(data,group)
This will return a list of 3 containing statistical significance(p-values) for enrichment, Fold change based on mean and Fold change based on median for the defined groups. In UniPath for downstream analysis, median fold change is considered.
Differential co-occurrence pathway analysis
Two cell populations can have differences in multiple ways. UniPath introduced the notion of differential co-occurrence pathway analysis for comparing two cell population. It is meant for studying mechanisms involved in controlling different properties of cells. For this purpose it has a function difcoccur() can be used where user can input pathway scores (raw adjusted p-values) and group.
For this tutorial, we have included raw adjusted pathway scores for lung cancer data which contains 162 cells. We are finding differentially co-occuring pathways in NSCLC TS cells with respect to Adherent NSCLC cells (88 to 162 cells).
library(UniPath)
##Differential cooccurence in NSCLC TS cells
##load lung cancer pathway scores data
data("lungCancer_pathway_scores")
##Define NSCLC TS cells in group 1
group = as.matrix(c(rep(1,87),rep(2,75)))
##Perform differential cooccurence
diff1 = difcoccur(pathwayscores,group)
##Two matrices will be generated
pval_TS = diff1$pval
diff_TS = diff1$dif
Similarly, we can find differentially co-occuring pathways in Adherent NSCLC cells with respect to TS NSCLC cells.
##Differential cooccurence in Adherent(Adh) NSCLC cells
data("lungCancer_pathway_scores")
##Define Adherent NSCLC cells in group 1
group = as.matrix(c(rep(2,87),rep(1,75)))
diff2 = difcoccur(pathwayscores,group)
pval_Adh = diff2$pval
diff_Adh = diff2$dif
For visualization extract relevant co-occuring pathway terms as shown in example below.
##Preprocessing of data in required for dotplot based visualization
##Based on the p-value and difference, we will visualize differential cooccurence using dotplot created using ggplot2
##Extract relevant cooccured pathway terms for TS cell group from both pvalue and difference files
pval_TSpathways = reshape2::melt(pval_TS[c("ST_WNT_BETA_CATENIN_PATHWAY","BIOCARTA_SHH_PATHWAY","KEGG_GLYCOLYSIS_GLUCONEOGENESIS"),c("KEGG_TGF_BETA_SIGNALING_PATHWAY","KEGG_NOTCH_SIGNALING_PATHWAY")])
pval_TSpathways = cbind.data.frame(paste(pval_TSpathways[,1],pval_TSpathways[,2],sep="-"),pval_TSpathways[,3])
diff_TSpathways = reshape2::melt(diff_TS[c("ST_WNT_BETA_CATENIN_PATHWAY","BIOCARTA_SHH_PATHWAY","KEGG_GLYCOLYSIS_GLUCONEOGENESIS"),c("KEGG_TGF_BETA_SIGNALING_PATHWAY","KEGG_NOTCH_SIGNALING_PATHWAY")])
diff_TSpathways = cbind.data.frame(paste(diff_TSpathways[,1],diff_TSpathways[,2],sep="-"),diff_TSpathways[,3])
##Defining group label
TS_group = c(rep("TS",6))
##Combine p-value,difference and group for relevant pathways in TS cells
TS = cbind.data.frame(pval_TSpathways,diff_TSpathways[,2],TS_group)
colnames(TS) = c("Pathways","Pvalue","diff","group")
##Same preprocessing in case of Adh cells as TS cells
##Extracting same pathways from ADh group from comparison with TS cells
pval_Adhpathways = reshape2::melt(pval_Adh[c("ST_WNT_BETA_CATENIN_PATHWAY","BIOCARTA_SHH_PATHWAY","KEGG_GLYCOLYSIS_GLUCONEOGENESIS"),c("KEGG_TGF_BETA_SIGNALING_PATHWAY","KEGG_NOTCH_SIGNALING_PATHWAY")])
pval_Adhpathways = cbind.data.frame(paste(pval_Adhpathways[,1],pval_Adhpathways[,2],sep="-"),pval_Adhpathways[,3])
diff_Adhpathways = reshape2::melt(diff_Adh[c("ST_WNT_BETA_CATENIN_PATHWAY","BIOCARTA_SHH_PATHWAY","KEGG_GLYCOLYSIS_GLUCONEOGENESIS"),c("KEGG_TGF_BETA_SIGNALING_PATHWAY","KEGG_NOTCH_SIGNALING_PATHWAY")])
diff_Adhpathways = cbind.data.frame(paste(diff_Adhpathways[,1],diff_Adhpathways[,2],sep="-"),diff_Adhpathways[,3])
Adh_group = c(rep("Adh",6))
Adh = cbind.data.frame(pval_Adhpathways,diff_Adhpathways[,2],Adh_group)
colnames(Adh) = c("Pathways","Pvalue","diff","group")
##Combine TS and Adh cell data
final = rbind(TS,Adh)
##Using ggplot2 we will plot a dotplot showing differentially cooccured pathways in TS and Adh cells
library(ggplot2)
p = ggplot() + geom_point(data=final, aes(x=group, y=Pathways, color=diff,size=Pvalue)) +
scale_color_gradient(low="red", high="blue") + scale_fill_gradient(low="grey", high="green") + scale_size(trans = 'reverse') + theme_light()
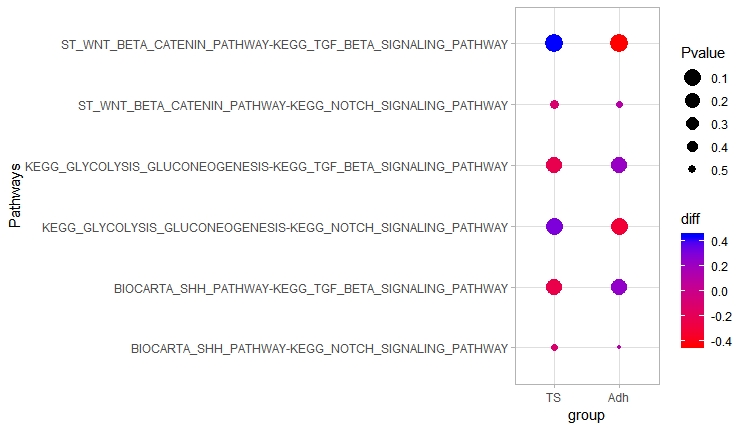
Above dot plot shows cooccurence of different pathway pairs in different cell types.
We have included some results for pseudo temporal ordering on simulated datastes which can be assessed using following link. Results for simulated datasets